AI in Medicine: Breakthrough Model Transforms Diagnosis
By Ella Coley, C2ST Intern, Waubonsee Community College
Imagine walking into a medical office and receiving a diagnosis in minutes rather than days. Researchers and biologists at Washington State University (WSU) have developed a new artificial intelligence (AI) model that has proven to help make this possible. This “deep learning” AI model examines tissue samples and identifies signs of disease on a microscopic level. It can potentially increase the speed of upcoming disease-related research and improve efficiency in medical diagnosis. WSU’s research team found that the model is both faster and more accurate than manual examination done by humans.
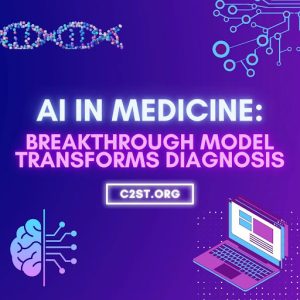
Colin Greeley, a former WSU graduate student, and his advising professor Lawrence Holder developed this AI model with tissue images from studies done by WSU biologist and co-corresponding author of the study, Michael Skinner. Using two elements: data preparation and the deep learning model, the researchers trained the AI to examine the tissue images and detect signs of disease efficiently.
The first element, data preparation, involves training the model to spot abnormalities. The research team did this using images with microscopic signs of disease in rats and mice’s kidneys, testes, ovaries, and prostates. The second element, the deep learning model, is a system that mimics the human brain “learning” from its mistakes. When the model makes an error in detection, it uses a process called backpropagation to make changes in the network so it won’t repeat the error.
By combining these two components, the WSU researchers developed the model to read extremely high-resolution images containing billions of pixels. Due to the size of the files, this AI model looks at detailed, individual sections that make a larger, lower-resolution image when put together, as if small tiles are arranged to form a mural. When this model was tested, the researchers found it performed extremely well.
Traditionally, tissue analysis for disease is a lengthy and detailed process, requiring teams of professionals to inspect and annotate tissue slides viewed under a microscope. Additionally, different examiners often check the slides several times to avoid errors, adding more to the process. When there are many slides to be examined, for example in research studies, collecting all the data can take over a year. With the advancement of this AI model to inspect human tissues, the process could be shortened from years to months or even weeks. This model is helpful even on a smaller scale when there aren’t many slides to research. Scientists describe that the model could improve the speed at which mammogram or MRI results are given. Skinner states, “It truly can be done in minutes… About 10 minutes rather than a day or two.” Additionally, the authors explain the model’s potential for improving diagnosis and research for cancer and other gene-related diseases.
Overall, this new AI model is a breakthrough in medical research and diagnosis. “The network that we’ve designed is state-of-the-art,” Holder said. “We did comparisons to several other systems and other data sets for this paper, and it beat them all.” With the progression and help of this model to diagnose disease in human samples, AI can help professionals optimize tissue examination and accurately find abnormalities that can be used to diagnose a patient. Researchers with WSU’s veterinary medicine are also interested in this model for diagnosing deer and elk tissue samples and studying emerging diseases in animals. With such a powerful tool on the rise, the future of diagnosis and research looks bright!
References:
- https://www.nature.com/articles/s41598-024-76807-x
- https://research.wsu.edu/news/ai-method-can-spot-potential-disease-faster-better-than-humans#:~:text=%E2%80%93%20A%20%E2%80%9Cdeep%20learning%E2%80%9D%20artificial,often%20more%20accurately%2C%20than%20people.
- https://www.kgw.com/article/news/health/ai-model-identifies-cancer-gene-pathology/283-3fe23b10-6bb6-4446-859d-a848ba53fe88